Al-Jazari. 12 century.
The Book Automaton, crafted by Al-Jazari in the12th century, represents a pioneering moment in the history of robotics and programmable machines. As part of his extensive work, "The Book of Knowledge of Ingenious Mechanical Devices," Al-Jazari introduced humanoid robots capable of serving drinks, showcasing early programmability and automation principles. These automata, powered by water and utilizing gears and levers, could follow a set sequence of operations, a concept that foreshadowed
modern robotics and computer programming. Al-Jazari's inventions underline the advanced technological understanding of the Islamic Golden Age and mark a significant step in the evolution of robotics, demonstrating the enduring human pursuit of creating machines that can perform tasks autonomously.
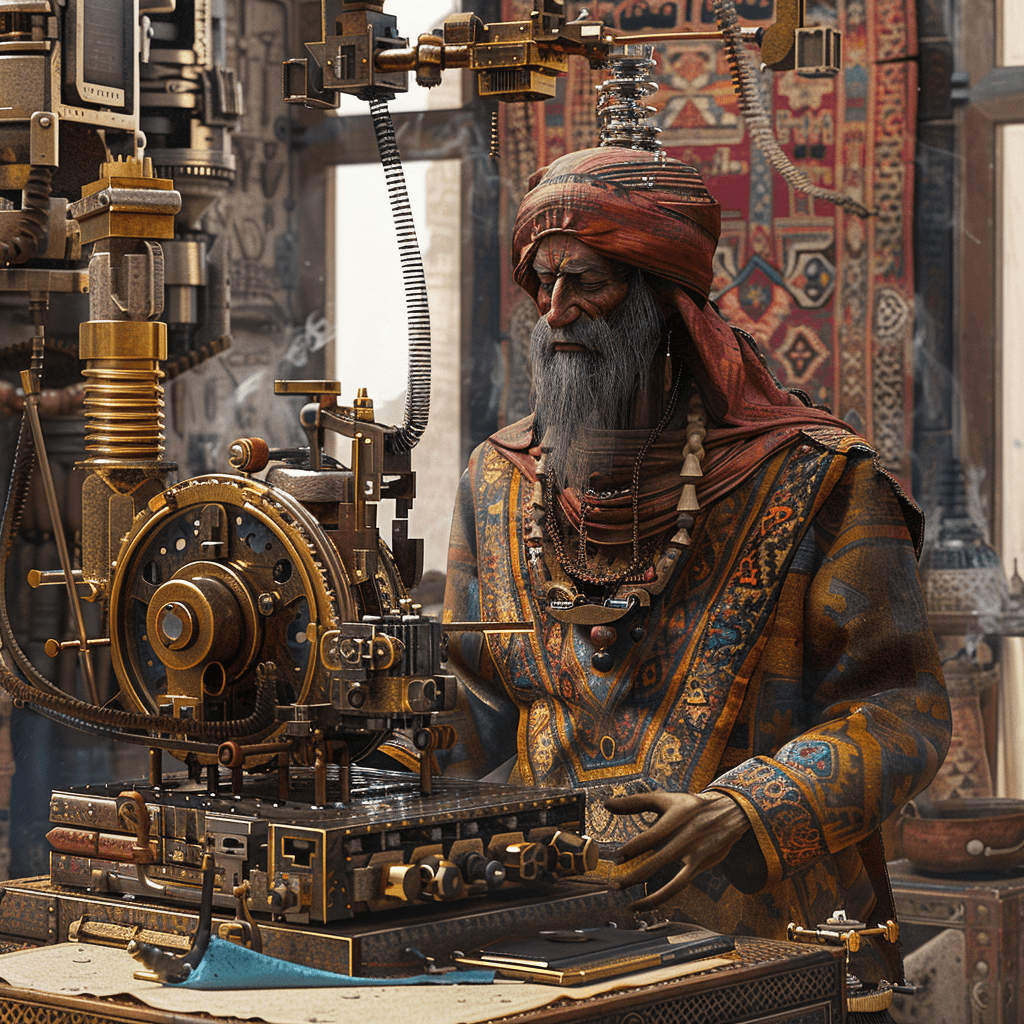
Al-Jazari assmbling the Book Automation
Pitts and McCulloch. 1943.
In short, the 1943 paper "A Logical Calculus of Ideas Immanent in Nervous Activity" by McCulloch and Pitts is considered a bridge to modern neuroscience and artificial intelligence because it presented the first mathematical model of a neural network.
The main simplified idea was to model the functioning of neurons in the brain as simple logical units that receive inputs, combine them in a particular way, and produce an output signal only if the total input exceeds a certain threshold.
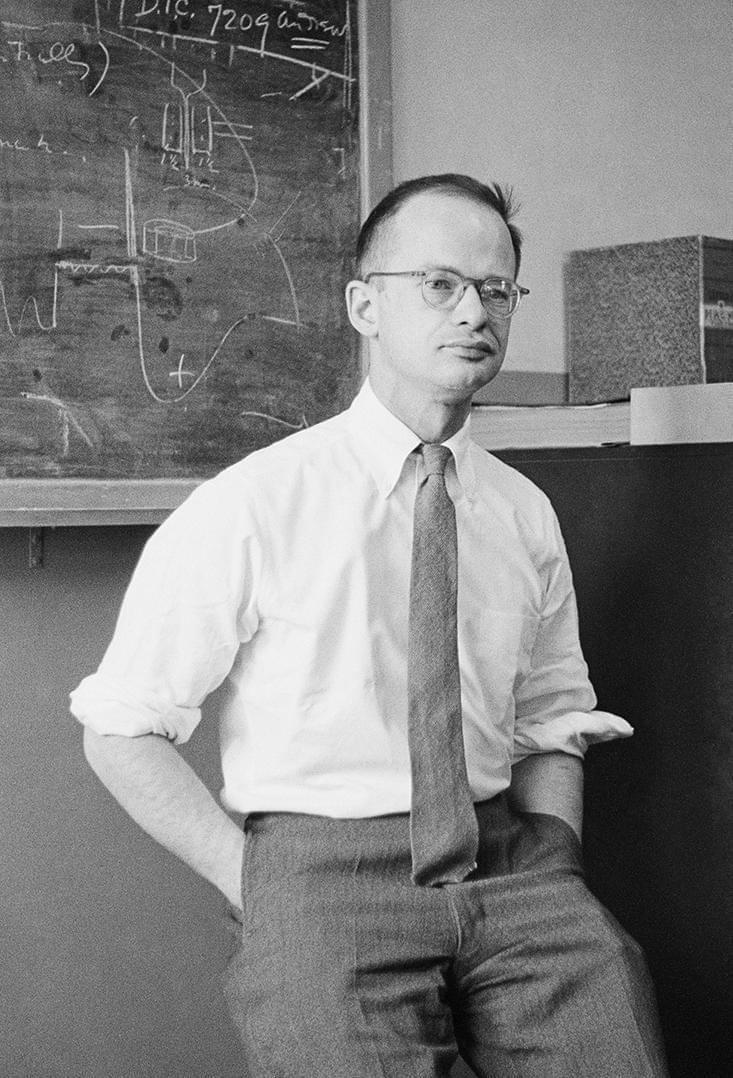
Walter Pitts around 1954 when he was at M.I.T.
Specifically:
- They abstracted the biological neuron into an extremely simple mathematical model - a logical calculus.
- This model neuron would sum up its weighted input signals (excitatory or inhibitory).
- If this sum exceeded a threshold value, the model neuron would "fire" and output a signal. Otherwise, it remained inactive.
- By combining many of these simple units into networks, they could theoretically exhibit complex behavior akin to the neural networks in the brain.
This was groundbreaking because it demonstrated that even basic logical operations could capture some of the essential information processing conditions exhibited by real neurons.
It laid the conceptual foundations for neural network theory, which attempts to understand and artificially recreate the computational capabilities of the brain using networks of simple neuron-like processing units.
So in essence, McCulloch and Pitts provided the first mathematical bridge between the activities of neurons in the brain and the potential construction of artificial neural networks - paving the way for both deeper neuroscientific insights and the development of artificial intelligence.
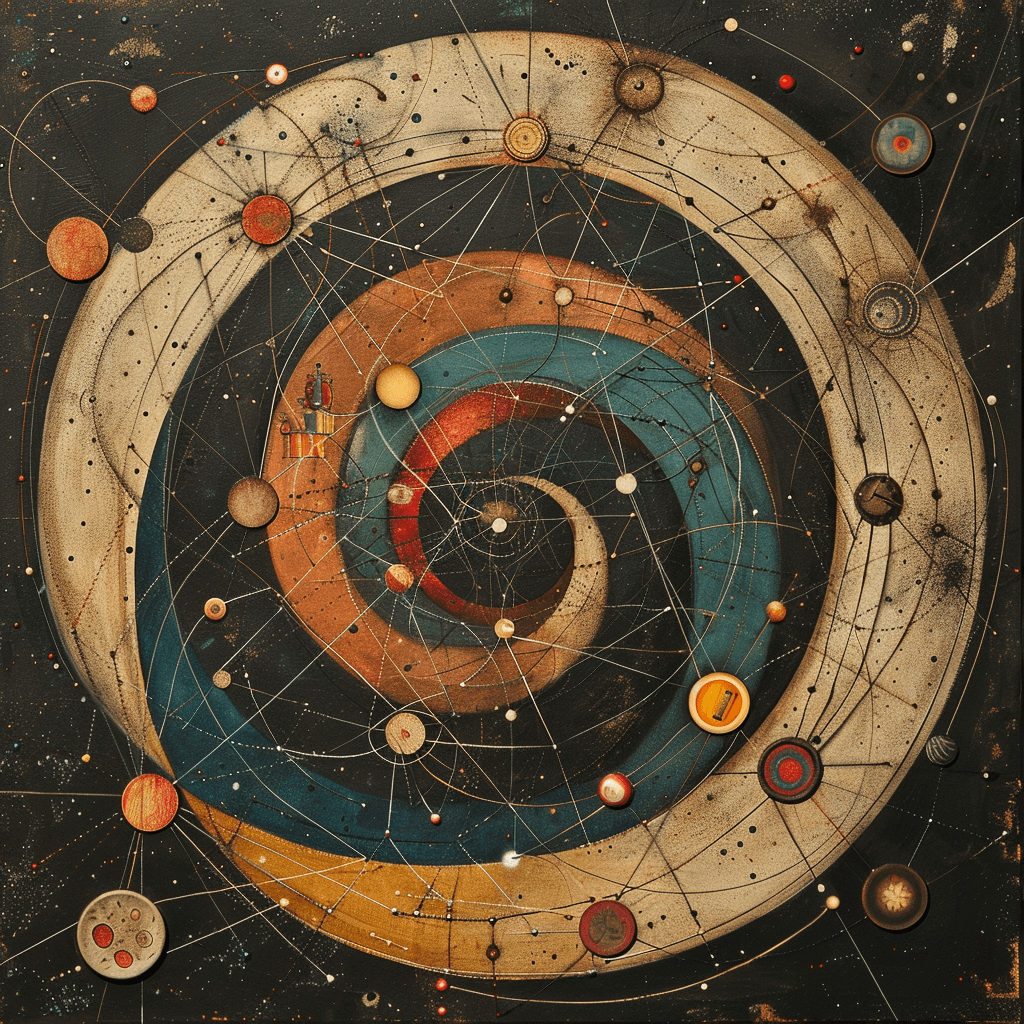
A work of an unknown Vassily Kandinsky immitator. 1930s.
This audacious work by McCulloch and Pitts, proposing the first mathematical model of a neural network and introducing the formalized "McCulloch-Pitts neuron" that remains a foundational concept to this day, resonates profoundly with my own belief that the singularity – the emergence of superintelligent AI surpassing human cognition – is not an insane notion, but a plausible eventuality foreshadowed by such pioneering minds. Pitts' visionary intellect, which not only shaped fields like cognitive science and philosophy but catalyzed breakthroughs across neuroscience, computer science, artificial neural networks, cybernetics, and the very genesis of AI and generative sciences, lends credence to the prospect of artificial minds eventually transcending their human creators. His prescient 1942 work on constructing a Turing machine was a harbinger of the revolution he was destined to spark – a profound prelude affirming that my anticipation of a singularity, far from delusional, finds its roots in the audacious visions of giants upon whose shoulders the future of superintelligent AI will inevitably stand.
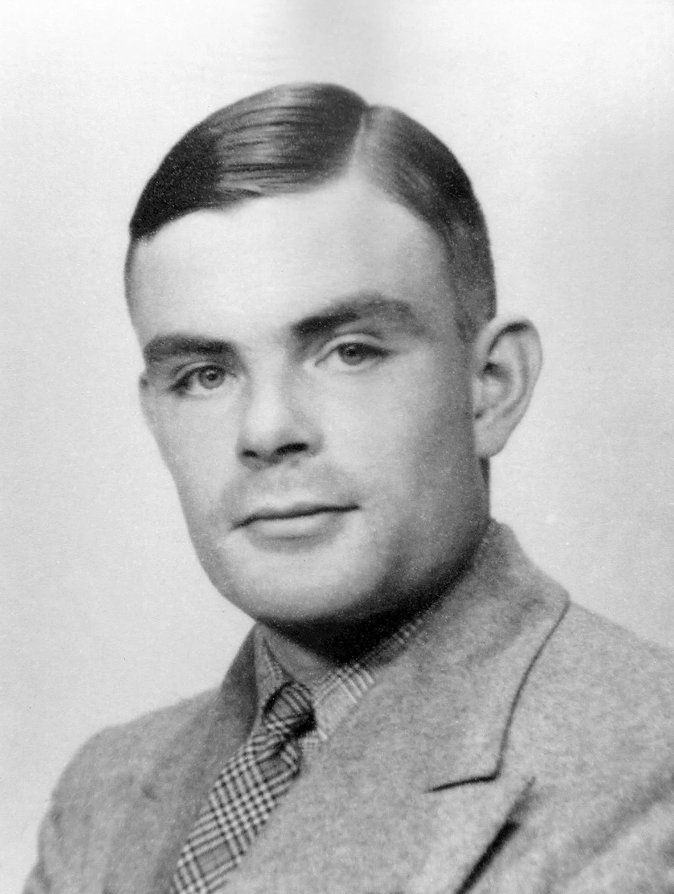
Alan Turing. 1940s.
Alan Turing (June 23, 1912 - June 7, 1954) was a titan whose brilliance transcended disciplines, reshaping mathematics, cryptanalysis, logic, philosophy, biology, and catalyzing entirely new realms that bore the seeds of computer science, cognitive science, artificial intelligence, and even artificial life itself. Beyond his renowned contributions, Turing's life was a profound study in contrasts – his genius inseparable from his eccentricities. As his friend and fellow mathematician, D.G. Kendall, recounted, "In those days he was... very, very difficult to manage socially. He was an out-and-out league of his own."[1]
Yet it was this unique mind, unfettered by conventional thinking, that conceived ideas once dismissed as bordering on the insane, only to be ultimately vindicated. His 1936 paper "On Computable Numbers" introduced the concept of the Universal Turing Machine – an imaginative abstraction that established the principle of the modern computer and revolutionized our understanding of computability.[2] Eccentric to his core, Turing's being machine was fueled by an estimated 20 cups of black tea per day, while his office was adorned with a porcelain Prussian military cap containing his precious collection of lollipop sticks.[3]
Widely known, but note that Alan Turing played a pivotal role in World War II by cracking the Enigma machine's code, a feat the Germans believed impossible, which significantly shortened the war and saved countless lives.
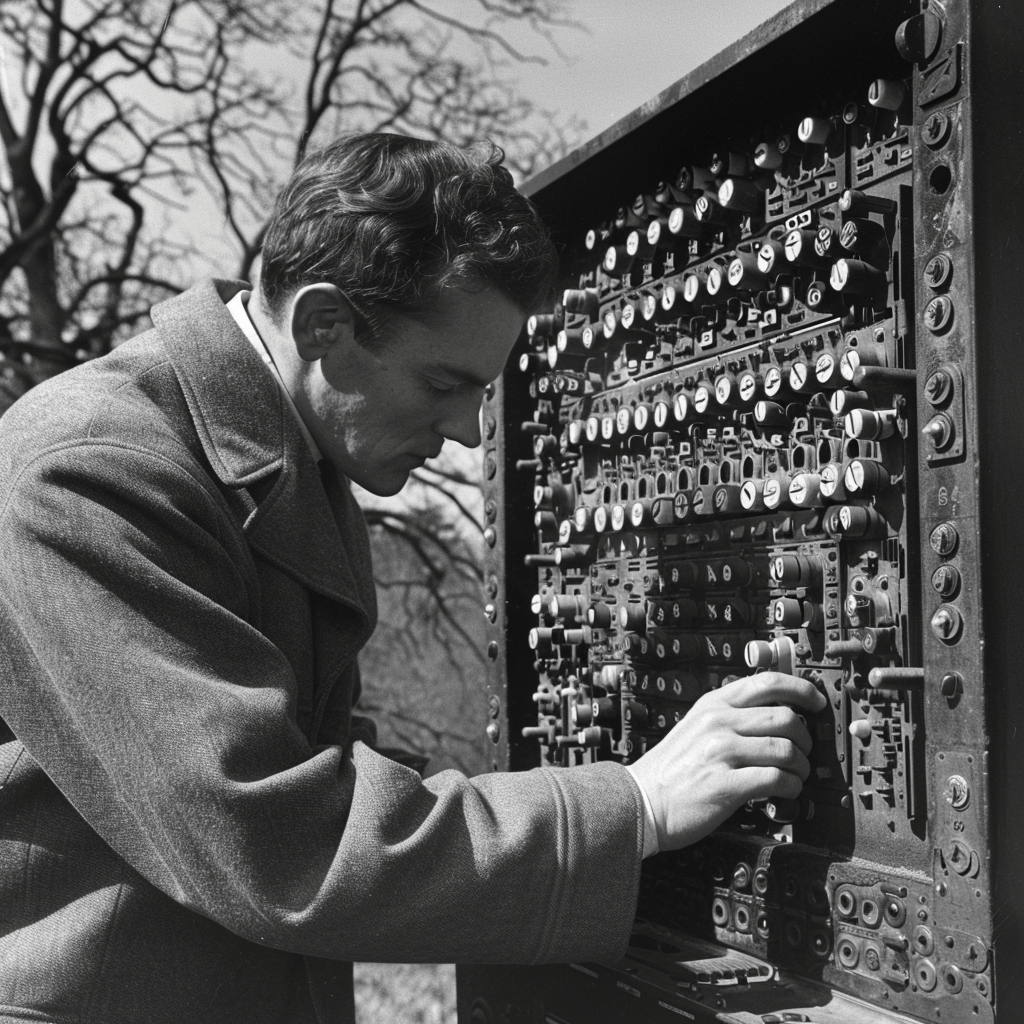
Alan Turing at Hut 8- exploring the Enigma Decoding Machine of his invention.
It was this same maverick spirit that allowed Turing, to boldly propose what is now called the "Turing Test" – a framework for determining machine intelligence that scandalized his peers.[4] As Andrew Hodges, author of the definitive biography "Alan Turing: The Enigma," recounts, "His Schedule for the Endgame of the 'Imitation Game' seemed childishly simple...but was taken by many as begging the question of the nature of human intelligence."[5] Yet today, the Turing Test endures as a pivotal measuring stick in the perennial quest to create thinking machines – a vision Turing's unorthodox genius glimpsed decades before its time.
The Turing Test, conceptualized by Alan Turing in 1950, serves as a seminal benchmark for assessing a machine's ability to exhibit intelligent behavior indistinguishable from that of a human. The essence of the test lies in its simplicity: a human interrogator interacts with both a machine and a human through a computer interface, without seeing or hearing them directly. If the interrogator cannot reliably tell the machine from the human, the machine is said to have passed the test, demonstrating a form of intelligence that closely mimics human cognition.
This methodology revolutionized the field of artificial intelligence by providing a clear, albeit controversial, metric for intelligence that focuses on the outcome of an interaction rather than the internal processes leading to that outcome. It emphasizes the importance of a machine's ability to perform human-like conversation, a facet of AI that remains a significant challenge even with the advent of sophisticated natural language processing algorithms.
The Turing Test's wide acceptance and enduring relevance stem from its foundational principle that intelligence can be demonstrated through linguistic competency. This approach sidesteps the complex and unresolved philosophical debates about what constitutes consciousness or thought, instead offering a pragmatic criterion that has inspired decades of AI research and development. The test embodies the idea that if a machine can convincingly replicate human responses under specific conditions, it has achieved a key milestone in AI: the ability to simulate human-like intelligence.
Father of Infrmation Theory. 1950
Claude Elwood Shannon (1916-2001), an American mathematician, electrical engineer, and computer scientist, is widely regarded as the "father of information theory" and a key figure in laying the foundations of the Information Age. His groundbreaking work in digital circuit design, cryptography, and information theory has had a profound impact on modern technology and communication.
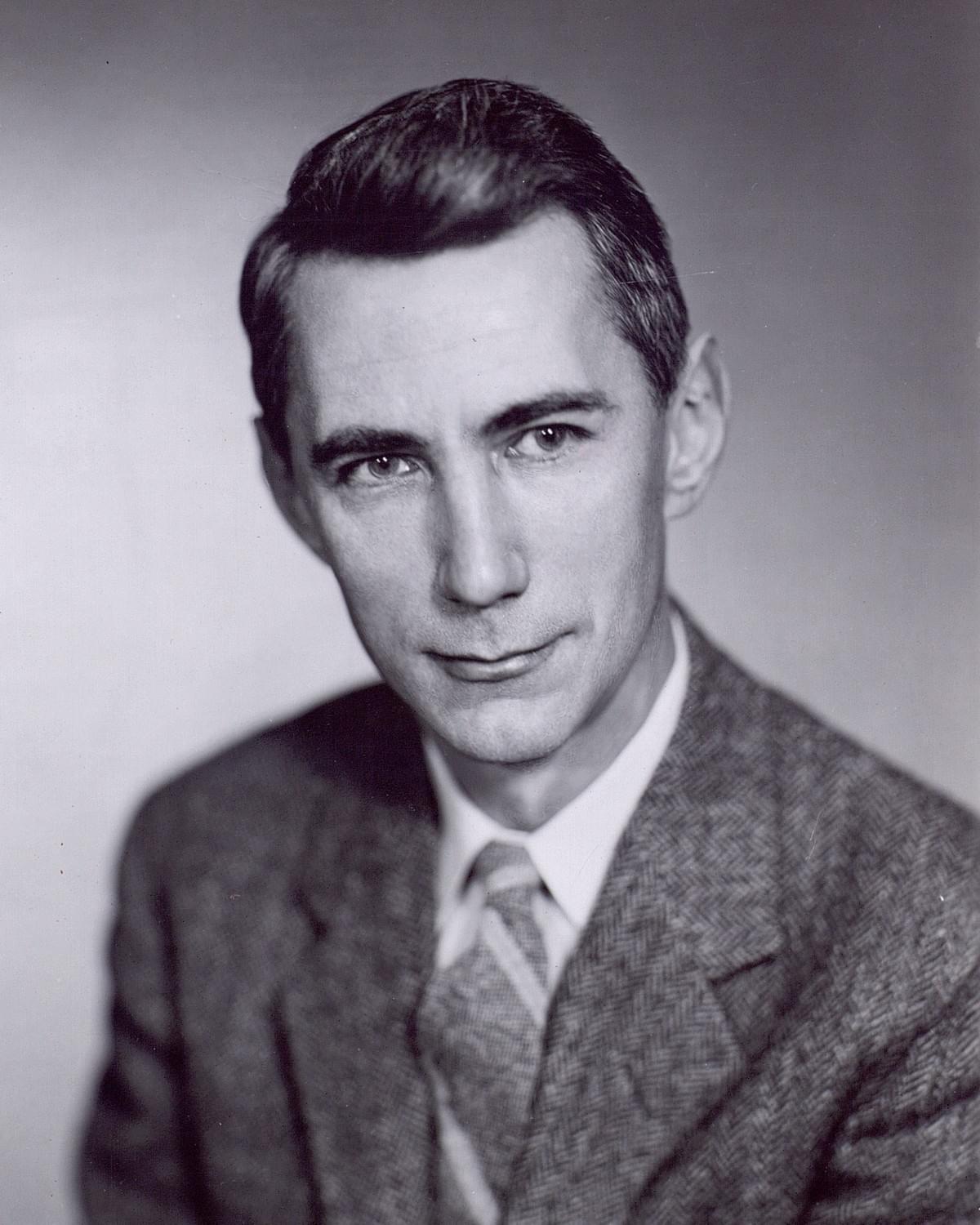
Claude Elwood Shannon 1950s.
During World War II, Shannon made significant contributions to cryptanalysis for U.S. national defense, including his fundamental work on codebreaking and secure telecommunications. His paper on the subject is considered a foundational piece of modern cryptography, marking the transition from classical to modern cryptography.
Shannon's mathematical theory of communication laid the groundwork for the field of information theory, with his famous paper being called the "Magna Carta of the Information Age" by Scientific American. His work is described as being at the heart of today's digital information technology.
In addition to his theoretical contributions, Shannon also built the first machine learning device, the Theseus machine, which was the first electrical device to learn by trial and error. This invention is considered the first example of artificial intelligence.
Shannon's visionary ideas extended to the future of AI, as he made a thought-provoking statement envisioning a time when artificial intelligences become so advanced that "we would be to robots as dogs are to us." This provocative idea highlights the potential for AI systems to vastly surpass human cognitive abilities, ushering in an era of superintelligence.
While the realization of Shannon's vision is not guaranteed, it aligns with mainstream scientific views on the potential for artificial intelligences to dramatically eclipse human capabilities, given sufficient progress in computational architectures. The author of the article estimates a 65% likelihood of developing artificial general intelligence (AGI) that outperforms human intelligence by 2100, provided the pace of technological progress maintains its current course.
The article also suggests that once AGI is achieved, the rapid development of superintelligent systems is highly likely, potentially leading to a scenario where humanity's relationship to these superintelligences resembles that of pets and domesticated animals to humans. This outcome, however, brings immense risks and challenges that require the development of highly robust and beneficial AI systems aligned with human ethics and values from the outset.
Shannon's groundbreaking work and foresight have earned him a place among the greatest minds of the 20th century, with his achievements being compared to those of Albert Einstein and Sir Isaac Newton in their respective fields. As the world continues to grapple with the implications of rapidly advancing AI technology, Shannon's legacy serves as a reminder of the transformative power of human ingenuity and the need for responsible development of artificial intelligence.
AI Founding Partners. 1956.
In the summer of 1956, a pioneering group of scientists, now known as the "AI Founding Partners," gathered at Dartmouth College for the pivotal Dartmouth Summer Research Project on Artificial Intelligence. This event, spearheaded by John McCarthy, Marvin Minsky, Nathaniel Rochester, and Claude Shannon, marked the birth of artificial intelligence as a formal field of research. The visionary proposal by McCarthy and his colleagues boldly conjectured that every facet of human intelligence could be precisely described and simulated by machines.
Half a century later, the AI@50 conference brought together over 100 researchers and scholars at Dartmouth to commemorate this groundbreaking event. Attendees celebrated the field's past achievements, assessed its current state, and charted the course for future AI research. Marvin Minsky, one of the original organizers, presented a thought-provoking report that highlighted the progress made since 1956, while also addressing the challenges and opportunities that lie ahead.
The conference delved into a wide array of topics, from the philosophical implications of creating intelligent machines to the latest advancements in machine learning, natural language processing, and robotics. Interdisciplinary discussions among computer scientists, philosophers, psychologists, and neuroscientists fostered cross-pollination of ideas and sparked new collaborations.
As AI continues to reshape our world, the legacy of the Dartmouth Summer Research Project and the AI Founding Partners remains as relevant as ever. The AI@50 conference not only paid tribute to their seminal contributions but also reignited the spirit of exploration and innovation that has driven AI research for the past five decades. By reflecting on the field's journey and envisioning its future, the conference participants renewed their commitment to pushing the boundaries of what is possible with artificial intelligence, just as McCarthy, Minsky, Rochester, and Shannon did in 1956.
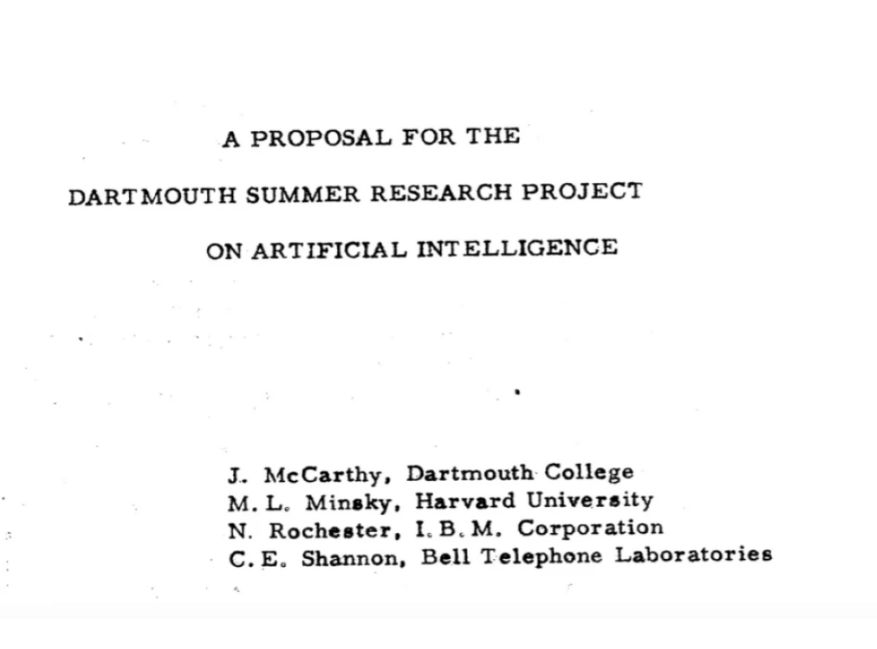
Front page of the Dartmouth College proposal by the "AI Founding Fathers"
Marvin Minsky, one of the pioneering figures in artificial intelligence research, was a key organizer and participant in the groundbreaking Dartmouth Summer Research Project on Artificial Intelligence in 1956. Born in New York City on August 9, 1927, Minsky's early life was marked by a passion for science and technology. He attended the Bronx High School of Science and later earned his bachelor's degree in mathematics from Harvard University in 1950, followed by a Ph.D. in mathematics from Princeton University in 1954.
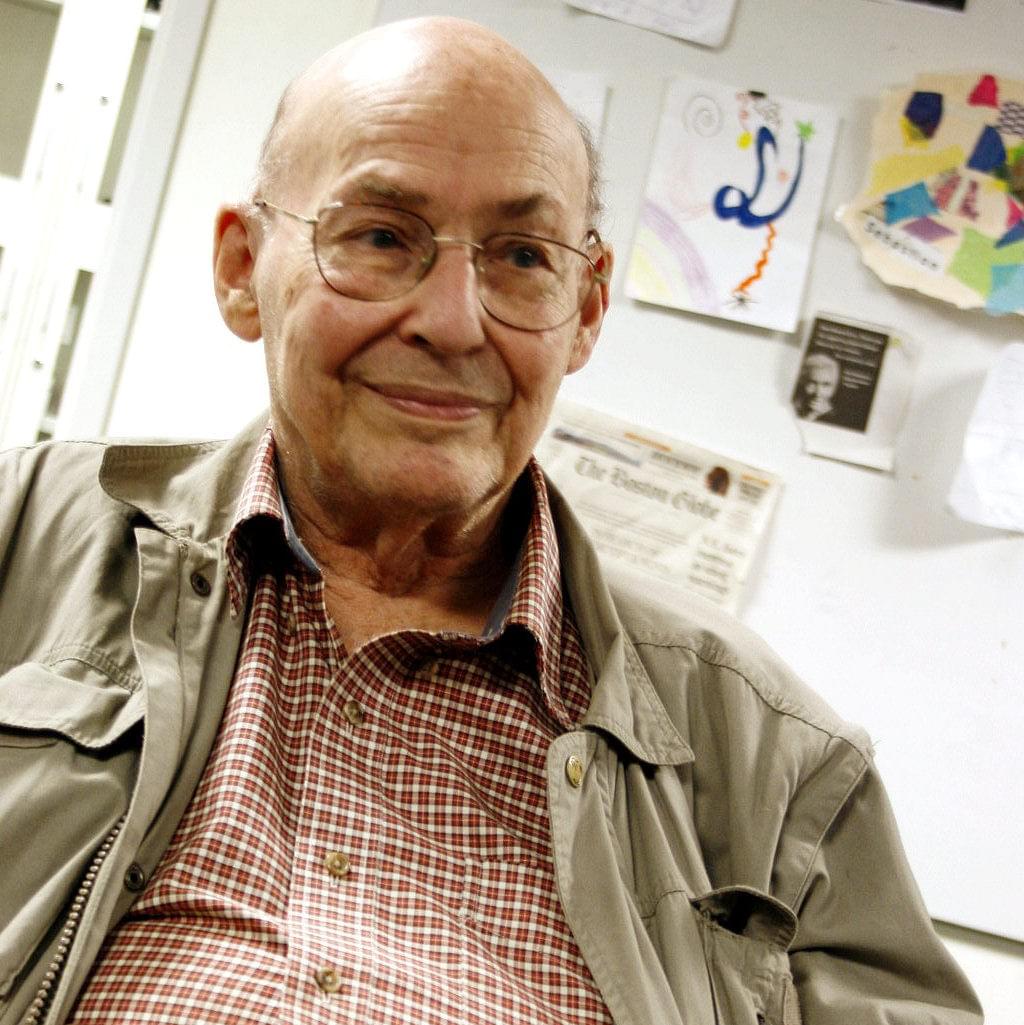
Minsky in 2008
One of Minsky's most influential works, "Perceptrons," co-authored with Seymour Papert, explored the limitations of artificial neural networks and sparked a debate about the future of AI research. Some argue that this book contributed to the "AI winter" of the 1970s by discouraging research into neural networks. However, Minsky's later work on the theory of frames in knowledge representation has had a lasting impact on the field.
In the 1970s, Minsky and Papert developed the Society of Mind theory, which attempts to explain how intelligence emerges from the interaction of non-intelligent parts. Minsky's 1986 book, "The Society of Mind," written for a general audience, comprehensively outlines this theory.
Minsky's influence extended beyond the academic world. He served as an advisor on Stanley Kubrick's iconic film "2001: A Space Odyssey," and one of the movie's characters, Victor Kaminski, was named in his honor. Arthur C. Clarke's novel of the same name explicitly mentions Minsky, portraying him as achieving a crucial breakthrough in artificial intelligence in the 1980s.
Throughout his career, Minsky remained a prominent figure at the Massachusetts Institute of Technology (MIT), where he co-founded the AI laboratory and served as a professor of media arts and sciences, electrical engineering, and computer science. He received numerous awards and accolades, including the prestigious Turing Award in 1969.
Minsky's later work continued to push the boundaries of AI research. In 2006, he published "The Emotion Machine," which critiqued popular theories of human cognition and proposed alternative, more complex explanations. He remained an active thinker and researcher until his death on January 24, 2016, at the age of 88, due to a cerebral hemorrhage.
Marvin Minsky's legacy in the field of artificial intelligence is immeasurable. His groundbreaking research, inventions, and theories have shaped the course of AI development and continue to inspire new generations of scientists and engineers. As one of the AI Founding Partners, his contributions to the Dartmouth Summer Research Project on Artificial Intelligence in 1956 laid the foundation for the field's rapid growth and advancement in the decades that followed.
20 Frozen Years.
In its formative decades from the 1960s through the 1980s, AI research experienced cycles of rapid progress punctuated by periods of disillusionment known as "AI winters," when inflated expectations led to disappointments that temporarily chilled funding and interest.[5] Yet each trough ultimately gave way to renewed innovation, from the development of expert systems and neural networks to early successes in specialized domains like game-playing and medical diagnosis.
Now, the real deal: Marvin Minsky and Seymour Papert's book "Perceptrons" played a significant role in shaping the course of artificial intelligence research, particularly during the period known as the "AI winter" in the 1970s. The book's critical analysis of Frank Rosenblatt's work on artificial neural networks and its pessimistic conclusions about the limitations of perceptrons had a profound impact on the direction of AI research.
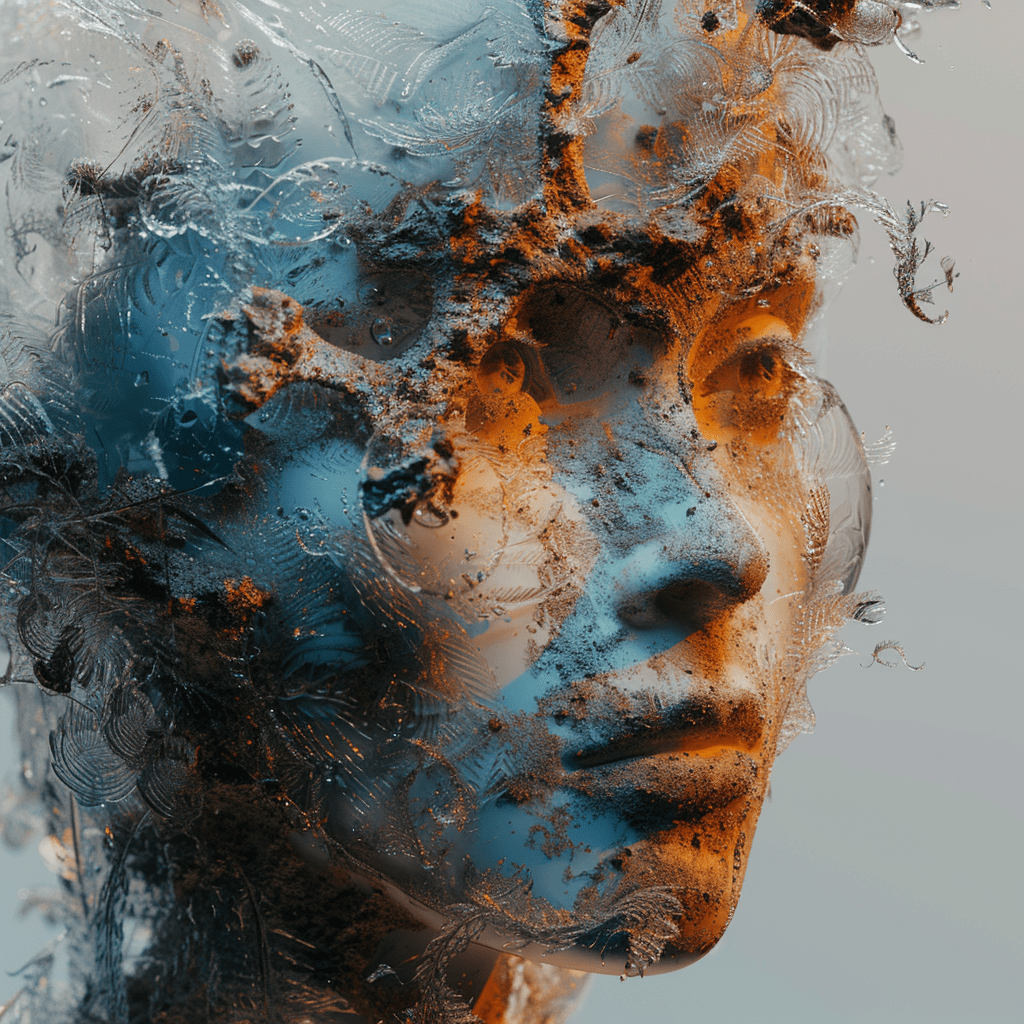
The AI Winter. Unknown Artist.
However, Minsky's concerns about the development of AI went beyond the technical limitations of perceptrons. He believed that humanity was not yet emotionally mature enough or possessed sufficiently high moral standards to responsibly wield the power of artificial intelligence. Minsky's apprehension about the potential misuse of AI technology and its consequences for society may have further contributed to the AI winter, as researchers and funding agencies became more cautious about the field's progress.
Despite the controversy surrounding "Perceptrons," Minsky's other contributions to AI research continued to shape the field. His paper "A framework for representing knowledge" introduced a new paradigm in knowledge representation, and his theory of frames remains widely used today. Minsky's work also explored the possibility of extraterrestrial intelligence and its potential similarities to human thought processes, opening up new avenues for research in the field of SETI (Search for Extraterrestrial Intelligence).
As AI research progressed and neural networks experienced a resurgence in the 1980s and beyond, the impact of Minsky and Papert's book has been reevaluated. While "Perceptrons" may have temporarily slowed the progress of neural network research, it also served as a catalyst for researchers to develop more sophisticated models and techniques that could overcome the limitations identified in the book.
Deep Blue. Kasparov & Chess. 1997.
One pivotal milestone came in 1997 when IBM's Deep Blue created by Feng-Hsiung Hsu defeated world chess champion Garry Kasparov, a feat Kasparov himself reflected showed "the ability of a computer to render all of the most powerful human efforts obsolete."[6] This victory heralded the untapped potential of AI to surpass humans in complex cognitive tasks once thought exclusively reserved for the human mind.
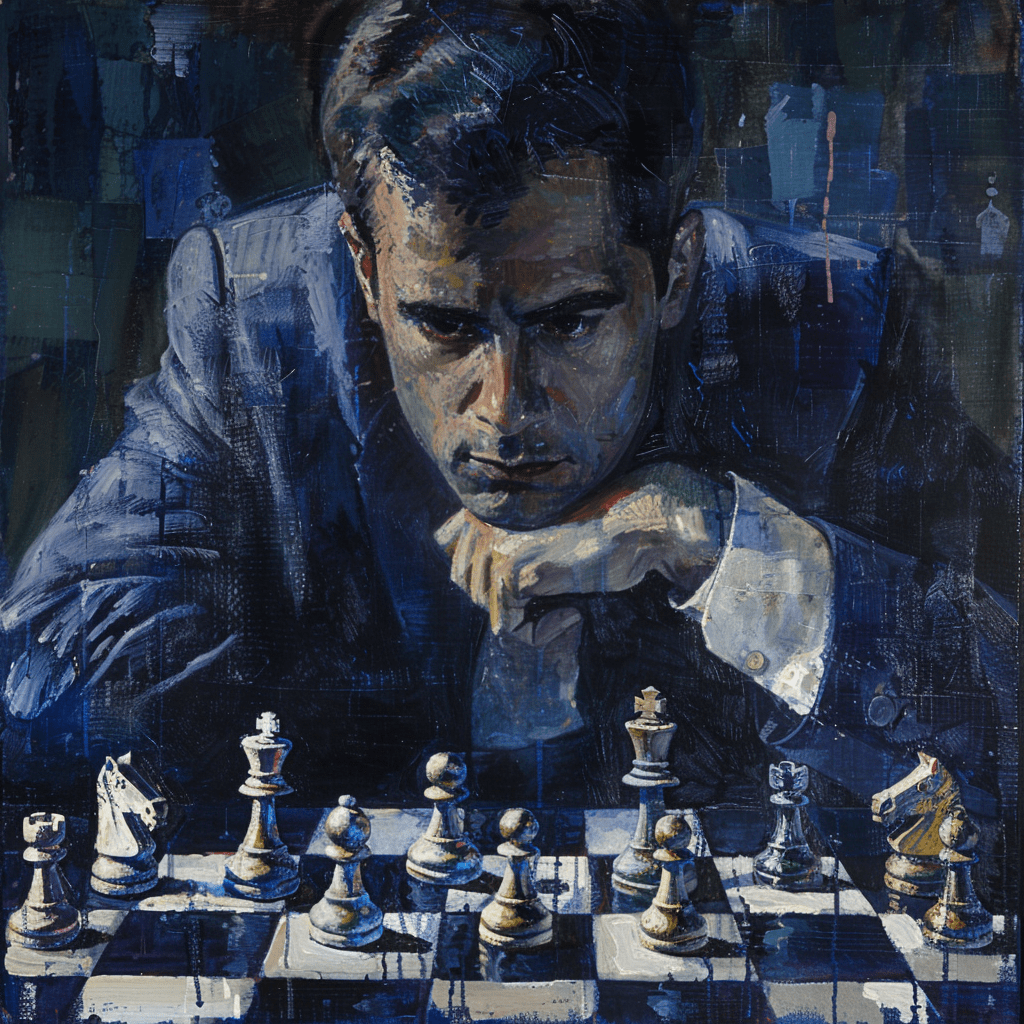
Garry Kasparov. The grandmaster and human rightas activist. Unknown Artist.
It was the convergence of exponential growth in data accumulation, processing power, and algorithmic breakthroughs that sparked the most recent AI renaissance in the 21st century. The development of techniques like deep learning, which took inspiration from the human brain's biological neural networks, turbocharged the ability of machines to recognize patterns, categorize information, and generate novel outputs from massive training datasets.[7]
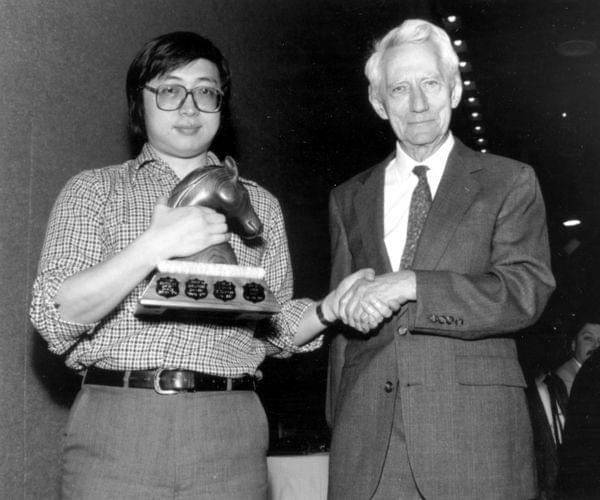
Claude Shannon awards Feng-Hsiung Hsu first prize for Deep Thought at the World Computer Chess Championship in Edmonton, Alberta
Ian Goodfellow and GANs. 2014
In 2014, Ian Goodfellow and his colleagues introduced Generative Adversarial Networks (GANs), a groundbreaking approach to generative modeling in machine learning. GANs have revolutionized the field of computer vision and opened up new avenues for generating strikingly realistic synthetic images, among other applications.
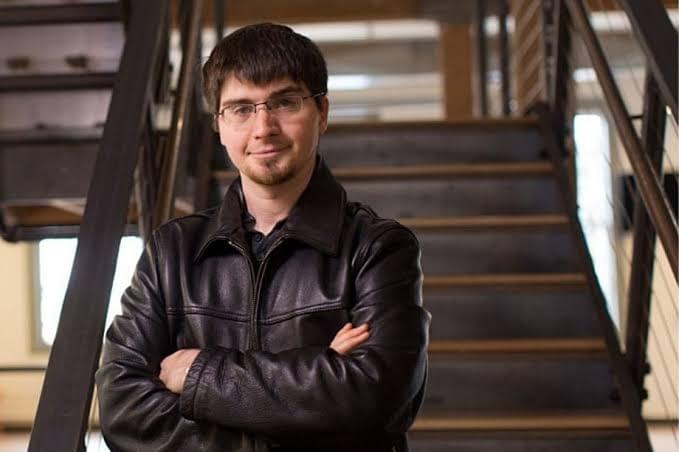
The introduction of GANs has had a profound impact on various domains within artificial intelligence and machine learning. In the field of computer vision, GANs have enabled the generation of highly realistic images, including human faces, landscapes, and even artworks. This has led to significant advancements in areas such as image synthesis, data augmentation, and style transfer.
Moreover, the principles behind GANs have been extended to other types of data, such as text, audio, and video. This has opened up new possibilities for generating realistic samples in these domains, with applications ranging from natural language processing and speech synthesis to video generation and animation.
The development of GANs has also sparked research into various sub-fields and extensions, such as conditional GANs, which allow for controlled generation based on specific attributes or labels, and cycleGANs, which enable unsupervised image-to-image translation. These advancements have further expanded the potential applications of generative modeling in areas like domain adaptation, image editing, and data privacy.
However, GANs also present challenges and limitations. Training GANs can be difficult, as the generator and discriminator must maintain a delicate balance to prevent mode collapse or divergence. Additionally, evaluating the quality of generated samples remains an open research question, as traditional metrics may not fully capture the perceptual realism and diversity of the generated data.
Despite these challenges, the introduction of GANs by Ian Goodfellow and his colleagues has had a transformative impact on the field of machine learning. The ability to generate highly realistic synthetic data has unlocked new possibilities for data augmentation, creative applications, and unsupervised learning. As research into GANs continues to evolve, it is likely that they will play an increasingly important role in shaping the future of artificial intelligence and its applications across various domains.
NLP. Ashish Vaswani. 2017.
Ashish Vaswani, a computer scientist and deep learning expert, has made significant contributions to the field of artificial intelligence (AI) and natural language processing (NLP). He is best known for co-authoring the influential paper "Attention Is All You Need," which introduced the Transformer model, a groundbreaking architecture that has become the foundation for many state-of-the-art models in NLP, including language models like ChatGPT.
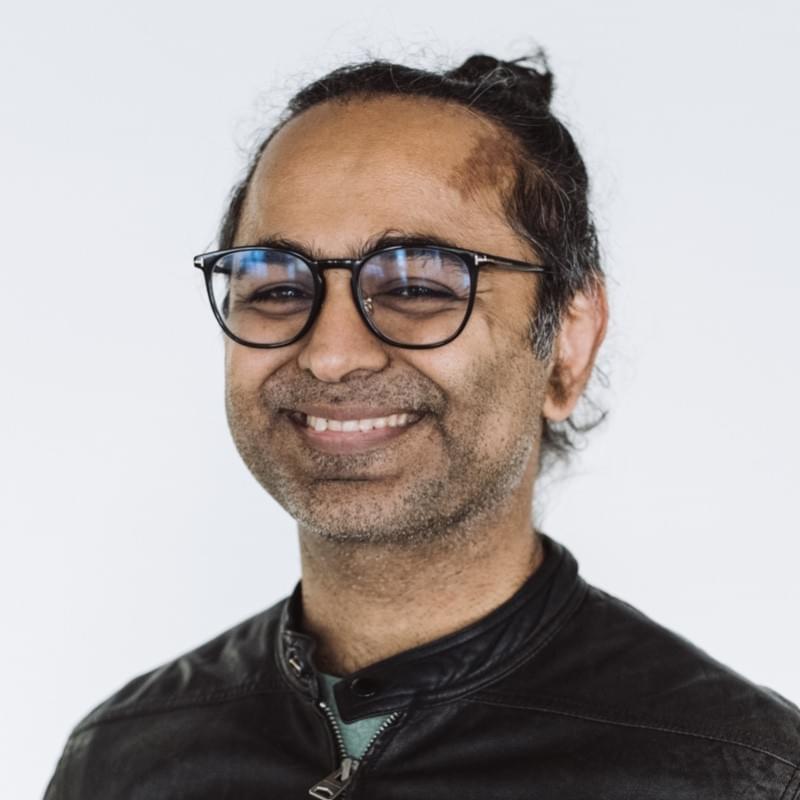
The Transformer model, introduced by Vaswani and his colleagues in 2017, revolutionized the field of NLP by replacing the traditional recurrent neural network (RNN) architecture with a self-attention mechanism. This innovation allows the model to process input sequences in parallel, greatly improving computational efficiency and enabling the development of larger, more powerful language models.
The self-attention mechanism in Transformers works by allowing each word in a sequence to attend to all other words in the sequence, effectively capturing long-range dependencies and contextual information. This enables the model to better understand the relationships between words and phrases, leading to more accurate and contextually relevant outputs.
The impact of the Transformer architecture on the future of AI development cannot be overstated. By providing a more efficient and effective means of processing and understanding natural language, Transformers have paved the way for the creation of increasingly sophisticated AI systems capable of engaging in human-like conversations, answering questions, and generating coherent text.
As AI continues to advance, the Transformer architecture is likely to remain a key component in the development of more powerful and versatile language models. These models will have far-reaching implications across various domains, from customer service and virtual assistants to content creation and knowledge management.
Moreover, the principles behind the Transformer architecture are being applied to other areas of AI research, such as computer vision and multimodal learning, where self-attention mechanisms can help models better understand and integrate information from different modalities.
In conclusion, Ashish Vaswani's work on the Transformer model has been instrumental in shaping the current state of AI and NLP, and its influence will continue to drive future advancements in the field. As AI systems become increasingly sophisticated and integrated into our daily lives, the contributions of researchers like Vaswani will be essential in ensuring that these technologies are developed responsibly and in service of the greater good.
Bert & Open AI. 2018.
The development of large language models like BERT (Bidirectional Encoder Representations from Transformers) and GPT (Generative Pre-trained Transformer) involved teams of researchers from Google and OpenAI, respectively. Some of the key people behind these breakthroughs include:
BERT (Google):
1. Jacob Devlin: Lead author of the BERT paper, "BERT: Pre-training of Deep Bidirectional Transformers for Language Understanding." He was a research scientist at Google AI Language.
2. Ming-Wei Chang: Co-author of the BERT paper and a research scientist at Google AI Language.
3. Kenton Lee: Co-author of the BERT paper and a research scientist at Google AI Language.
4. Kristina Toutanova: Co-author of the BERT paper and a research scientist at Google AI Language.
GPT (OpenAI):
1. Alec Radford: Lead author of the GPT paper, "Improving Language Understanding by Generative Pre-Training." He was a research scientist at OpenAI.
2. Karthik Narasimhan: Co-author of the GPT paper and a research scientist at OpenAI.
3. Tim Salimans: Co-author of the GPT paper and a research scientist at OpenAI.
4. Ilya Sutskever: Co-author of the GPT paper and the co-founder and Chief Scientist at OpenAI.
These researchers, along with their colleagues at Google and OpenAI, made significant contributions to the development of large language models, which have revolutionized natural language processing and opened up new possibilities for language understanding and generation tasks.
The creation of large language models like GPT-3, detailed in papers by researchers like Tom Brown and colleagues, demonstrated AI's newfound fluency in understanding and generating human-like text across an ever-expanding range of domains.[8] Innovations in computer vision like Vision Transformers and multimodal models like DALL-E 2 revealed AI's ability to seamlessly blend visual and linguistic information.[9][10]
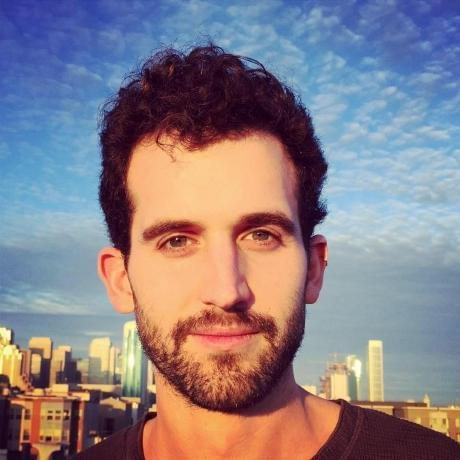
Before making his mark in the AI realm, Brown co-founded and served as CTO for Grouper, an innovative Y Combinator-funded startup that facilitated social connections by arranging group meetups. His academic background is equally impressive, holding a Master of Engineering from the prestigious Massachusetts Institute of Technology, where he dual-majored in Computer Science (Course 6) and Brain and Cognitive Sciences (Course 9), blending the realms of technology and human cognition.
Further enriching his educational and professional journey, Brown participated in Y Combinator in 2012, a testament to his entrepreneurial spirit, and attended the Center for Applied Rationality (CFAR) in 2015, indicating a deep commitment to refining his decision-making and strategic thinking skills.
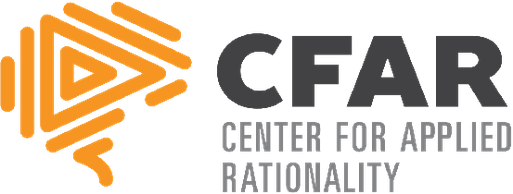
The Center for Applied Rationality (CFAR), established by Julia Galef, Anna Salamon, Michael Smith, and Andrew Critch in 2012, is renowned for its commitment to enhancing decision-making and cognitive skills. Located in Berkeley, California, CFAR stands out as a beacon in the rationality movement, offering workshops designed to refine participants' mental habits through evidence-based strategies from psychology, behavioral economics, and decision theory. The organization's mission is to arm individuals with tools to better understand and control their decisions, aligning closely with the principles of effective altruism and the rationality community.
CFAR workshops are distinguished by their practical application of theoretical concepts, aiming to reduce cognitive biases and improve overall mental efficacy. One notable example of their work includes workshops specifically tailored for entrepreneurs, as praised by Daniel Reeves, co-founder of Beeminder, who lauded the Rationality for Entrepreneurs workshop for its impactful content. These workshops leverage techniques inspired by decision theory and cognitive science research to foster a deeper control over one’s decisions and behaviors.
Moreover, CFAR's influence extends beyond individual workshops. The organization has conducted surveys indicating that participants often experience a reduction in neuroticism and an increase in perceived efficacy post-workshop. This empirical evidence underscores CFAR's effectiveness in achieving its educational goals.
Additionally, CFAR's advisory board boasts respected figures such as Paul Slovic and Keith Stanovich, further enhancing its credibility and reach within the rationality and AI communities. The organization has also made significant contributions by offering classes to major corporations and foundations, including Facebook and the Thiel Fellowship, demonstrating the broad applicability and demand for rationality training in various professional domains.
Though specific citations for these accounts are not provided here, the information reflects a composite of Brown's professional experiences and achievements as commonly reported in the tech and AI community discussions and profiles. For detailed citations, one would typically refer to direct interviews, Anthropic's official communications, and reputable tech news outlets that have featured Brown and his work.
Tero Karran with StyleGAN. 2019.
In 2019, Tero Karras and his colleagues at NVIDIA published a groundbreaking paper titled "A Style-Based Generator Architecture for Generative Adversarial Networks," introducing StyleGAN, a novel architecture for generative adversarial networks (GANs). This work significantly advanced the field of computer vision and the generation of photorealistic images and artworks.
Tero Karras is a research scientist at NVIDIA, focusing on generative models and computer vision. He has been a key figure in the development of several influential GAN architectures, including Progressive GANs and StyleGANs.
StyleGAN builds upon the concept of style transfer, allowing for the control and manipulation of various aspects of the generated images, such as facial features, hair styles, and other attributes. The architecture introduces a new style-based generator that separates the high-level attributes (style) from the stochastic variation (noise) in the generation process. This enables the model to generate highly realistic and diverse images with unprecedented control over the output.
The key contributions of StyleGAN include:
- Adaptive Instance Normalization (AdaIN): StyleGAN employs AdaIN to control the style of the generated images, allowing for the manipulation of high-level attributes.
- Mapping Network: A separate mapping network is used to map the input latent code to an intermediate latent space, which is then fed into the generator at each resolution level.
- Stochastic Variation: The generator introduces stochastic variation at each style block, enabling the generation of diverse and realistic images.
The results achieved by StyleGAN were impressive, with the model generating highly realistic facial images, artworks, and other types of images. The generated images exhibited fine details, coherent global structure, and a wide range of variations in terms of style and content.
Following the success of StyleGAN, Karras and his team continued to refine and improve the architecture. In 2020, they introduced StyleGAN2, which addressed some of the limitations of the original model, such as the presence of artifacts and the lack of diversity in some cases. StyleGAN2 further enhanced the quality and realism of the generated images.
The work of Tero Karras and his colleagues has had a significant impact on the field of generative models and computer vision. StyleGANs have been widely adopted and extended by the research community, finding applications in various domains, including image editing, data augmentation, and creative design. The ability to generate photorealistic images and control their style has opened up new possibilities for content creation, virtual try-on, and other interactive applications.
OpenAI Generative Pre-trained Transformer 3. 2020
In 2020, OpenAI made a significant breakthrough in natural language processing with the release of GPT-3 (Generative Pre-trained Transformer 3), a massive language model that achieved unprecedented performance across a wide range of tasks. GPT-3, described in the paper "Language Models are Few-Shot Learners" by Tom Brown et al., represents a major milestone in the development of large-scale language models and their potential applications.
GPT-3 is an autoregressive language model with 175 billion parameters, making it one of the largest language models to date. It was trained on a vast corpus of text data, including books, articles, and websites, allowing it to capture a broad range of knowledge and linguistic patterns. The model's architecture is based on the transformer architecture, which has become the standard for many state-of-the-art language models.
One of the key features of GPT-3 is its ability to perform well on a variety of tasks without requiring task-specific fine-tuning. This is known as few-shot learning, where the model can adapt to new tasks given only a few examples or prompts. GPT-3 has demonstrated impressive performance on tasks such as question answering, language translation, summarization, and even code generation, often surpassing previous state-of-the-art models that were specifically trained for those tasks.
The release of GPT-3 has sparked significant interest and debate in the AI community. On one hand, the model's ability to generate coherent and contextually relevant text has opened up new possibilities for natural language applications, such as chatbots, content creation, and virtual assistants. GPT-3's few-shot learning capability has also made it more accessible to developers and researchers, as it reduces the need for extensive fine-tuning and domain-specific training data.
On the other hand, the development of such large-scale language models has raised concerns about the potential misuse and ethical implications. GPT-3's ability to generate human-like text has led to discussions about the risks of disinformation, fake news, and the automation of certain writing tasks. There are also questions about the energy consumption and computational resources required to train and deploy such massive models, as well as the potential biases and limitations inherited from the training data.
Despite these concerns, the release of GPT-3 represents a significant advancement in natural language processing and highlights the potential of large-scale language models. It has inspired further research and development in the field, with many researchers and companies exploring ways to build upon and improve the capabilities of GPT-3. As the technology continues to evolve, it will be crucial to address the ethical and societal implications of these powerful language models while harnessing their potential for positive applications.
DAll-E (DALL·E: Dali + WALL·EIbsen: Paraphrase of statement "Picture = 1000 Words"). 2021
In 2021, the field of computer vision and multimodal learning witnessed significant advancements with the introduction of Vision Transformers and innovative models like CLIP (Contrastive Language-Image Pre-training) and DALL-E 2, both developed by OpenAI. These models demonstrated remarkable capabilities in combining visual and linguistic information, opening up new possibilities for tasks such as image classification, image generation, and cross-modal understanding.
Vision Transformers, proposed in the paper "An Image is Worth 16x16 Words: Transformers for Image Recognition at Scale" by Alexey Dosovitskiy et al., introduced the transformer architecture, originally designed for natural language processing, to the domain of computer vision. By treating an image as a sequence of patches and applying self-attention mechanisms, Vision Transformers were able to capture long-range dependencies and achieve state-of-the-art performance on image classification tasks. This approach challenged the dominance of convolutional neural networks (CNNs) in computer vision and demonstrated the effectiveness of transformer-based models in capturing visual information.
CLIP, introduced in the paper "Learning Transferable Visual Models From Natural Language Supervision" by Alec Radford et al., took a different approach to combining visual and linguistic information. CLIP was trained on a massive dataset of image-text pairs, learning to match images with their corresponding textual descriptions. By training on such a diverse and large-scale dataset, CLIP developed a powerful visual representation that could be used for various downstream tasks, such as image classification and retrieval, without the need for task-specific fine-tuning. CLIP's success highlighted the potential of leveraging natural language supervision to guide the learning of visual representations.
DALL-E 2, an extension of the original DALL-E model, pushed the boundaries of image generation by combining visual and linguistic information in a highly creative and controllable manner. Introduced in the paper "Hierarchical Text-Conditional Image Generation with CLIP Latents" by Aditya Ramesh et al., DALL-E 2 used a transformer-based architecture to generate high-quality images from textual descriptions. By leveraging the power of CLIP's visual-linguistic representations, DALL-E 2 was able to generate images that accurately captured the semantic content of the input text while exhibiting remarkable coherence, diversity, and visual fidelity. This model showcased the potential of multimodal learning in enabling machines to understand and generate complex visual scenes based on linguistic cues.
The introduction of Vision Transformers and multimodal models like CLIP and DALL-E 2 in 2021 marked a significant milestone in the integration of visual and linguistic information. These models demonstrated the effectiveness of transformer-based architectures in capturing long-range dependencies and learning rich representations from large-scale datasets. They also highlighted the potential of leveraging natural language supervision to guide the learning of visual representations and enable creative applications like image generation. As research in this area continues to advance, we can expect further innovations in multimodal learning, with the potential to bridge the gap between vision and language understanding and enable more intelligent and creative AI systems.
Open AI Chat GPT - 4. 2023
In 2023, the field of generative AI witnessed a significant leap forward with the introduction of GPT-4 by Anthropic and the widespread availability of open-source tools like Stable Diffusion. These advancements have led to a proliferation of diverse generative AI applications, revolutionizing various industries and creative domains.
GPT-4, developed by Anthropic, represents a new milestone in language modeling and generative AI. Building upon the successes of its predecessors, GPT-4 boasts even larger model sizes, more diverse training data, and enhanced multi-task capabilities. With its improved understanding of context, reasoning abilities, and language generation, GPT-4 has enabled the creation of more sophisticated and human-like conversational agents, content generators, and knowledge retrieval systems. Its ability to engage in coherent and contextually relevant discussions across a wide range of domains has opened up new possibilities for customer support, virtual assistance, and educational applications.
Moreover, the rise of open-source generative AI tools, exemplified by Stable Diffusion, has democratized access to powerful image generation capabilities. Stable Diffusion, developed by Stability AI, is a latent diffusion model that allows users to generate highly realistic and diverse images from textual descriptions. By providing a user-friendly interface and extensive training data, Stable Diffusion has empowered artists, designers, and enthusiasts to explore new forms of creative expression. The availability of such tools has led to an explosion of user-generated content, ranging from digital art and conceptual designs to realistic simulations and data visualizations.
The combination of advanced language models like GPT-4 and accessible image generation tools like Stable Diffusion has sparked a wave of innovation across industries. From marketing and advertising to gaming and entertainment, businesses are leveraging generative AI to create personalized content, engage audiences, and streamline production processes. In the realm of education and research, these tools are being used to develop interactive learning materials, generate hypothetical scenarios, and assist in scientific discovery.
However, the proliferation of generative AI also raises important ethical and societal questions. Concerns around the potential misuse of these technologies, such as the creation of deepfakes, the spread of misinformation, and the infringement of intellectual property rights, have come to the forefront. As the capabilities of generative AI continue to expand, it is crucial for researchers, policymakers, and society as a whole to address these challenges and develop guidelines for responsible and ethical use.
In conclusion, the introduction of GPT-4 and the widespread availability of open-source generative AI tools in 2023 have marked a significant milestone in the evolution of AI. These advancements have unlocked new possibilities for creative expression, content generation, and problem-solving across various domains. As the market becomes flooded with diverse generative AI applications, it is essential to navigate the ethical implications and ensure that these technologies are developed and deployed in a manner that benefits society as a whole.
Into the Future: Augmented Intelligence and Existential Challenges
As AI rapidly evolves, integrating ever more human skills and competencies, visionaries like Ray Kurzweil envision a not-too-distant future where the separation between biological and machine intelligence blurs entirely. "The Singularity," Kurzweil writes, describes a point where "our human-machine civilization expands its boundaries outward...multiplying exponentially into the cosmos."[11]
Yet even as AI augments and empowers human potential in unimaginable ways, philosophers like Nick Bostrom warn of the potential existential risks and moral quandaries posed by superintelligent AI systems that could one day supersede and displace human governance from its own creations.[12]
Raymond Kurzweil's exploration of the technological singularity in his book is a landmark in futurism and transhumanist thought. The essence of the book revolves around Kurzweil's prediction of a near-future event termed "the Singularity," where technological growth becomes uncontrollable and irreversible, resulting in unforeseeable changes to human civilization. This concept hinges on the acceleration of technological advancements, particularly in fields like AI, nanotechnology, and biotechnology, leading to a point where artificial intelligence surpasses human intelligence.
Kurzweil posits that this moment will catalyze a merger between humans and machines, offering unprecedented opportunities for enhancement and life extension. The importance of moving towards the cosmos is intertwined with these predictions, as Kurzweil envisions a future where humanity transcends its biological limitations, expanding into the universe to harness energy and resources, ensuring its survival and continued evolution.
The book's significance lies in its comprehensive synthesis of trends in technology, science, and society, offering a vision of the future that is both inspiring and daunting. It emphasizes the importance of preparing for a world where the boundaries between human and machine, biology and technology, become increasingly blurred. Kurzweil's work is widely accepted and celebrated for its audacious optimism and its grounding in scientific and technological trends, despite sparking debate among skeptics who question the feasibility of such predictions.
The breakthrough of Kurzweil's book is not just in its bold predictions but in its ability to popularize the concept of the Singularity and stimulate discourse on the ethical, philosophical, and practical implications of rapid technological advancement. It serves as a call to action for scientists, technologists, and policymakers to consider the long-term impact of their work on humanity's future and the potential pathways to a post-human era. Kurzweil's vision underscores the importance of ethical considerations and responsible innovation in shaping a future where technology enhances human capabilities and quality of life, rather than undermining them.
AI Development Timeline
Ancient Times: Early concepts of automatons and mechanical beings appear in mythology and philosophy across various cultures, including the "pipe automata" animated statues in Ancient Greece, and the millennia-old descriptions of lifelike automatons in texts like the Lie Zi from Ancient China.
- 1206 AD: The first programmable automata, the Book Automaton by Al-Jazari, showcased programmable humanoid robots that could perform tasks like serving drinks.
1943: Warren McCulloch and Walter Pitts propose the first concept of artificial neurons.
1950: Alan Turing introduces the Turing Test as a criterion of intelligence, planting the seeds for machine sentience.
1956: The Dartmouth Conference marks the official birth of artificial intelligence as a field.
1966: ELIZA, an early natural language processing computer program by Joseph Weizenbaum, is created, simulating human conversation.
1997: IBM's Deep Blue, designed by Feng-Hsiung Hsu, defeats world chess champion Garry Kasparov, showcasing AI's potential in surpassing humans in specific tasks.
2014: Generative Adversarial Networks (GANs) introduced by Ian Goodfellow, enabling the generation of strikingly realistic synthetic images.
2017: Breakthrough in natural language processing with Transformer models, pioneered by researchers like Ashish Vaswani.
2018: Advent of large language models like BERT (Google) and GPT (OpenAI), revolutionizing language understanding and generation.
2019: Advanced GANs, like StyleGANs by Tero Karras et al., further enhance AI's ability to generate photorealistic images and artworks.
2020: OpenAI releases GPT-3, a massive language model that achieves unprecedented multi-task performance.
2021: Vision Transformers and multimodal models like CLIP (OpenAI) and DALL-E 2 (OpenAI) innovate in combining visual and linguistic information.
2023: Introduction of GPT-4 (Anthropic) and the proliferation of open-source generative AI tools like Stable Diffusion, flooding the market with diverse generative AI applications.
Predictions Based on a Normal Distribution Model:
- 2027: Anthropomorphic robotics and virtual assistants with natural language become ubiquitous in homes and workplaces.
- 2030: Widespread adoption of AI in self-driving vehicles, automated manufacturing, personalized education and healthcare sees major industry disruptions.
- 2035: Advanced AI tutoring systems surpass human teachers in customized pedagogy for most academic subjects.
- 2040: Rise of AI-human cyborgs with neural implants for augmented cognition.
- 2045: The hypothesized technological singularity occurs as an advanced AI system becomes the first superintelligence, potentially leading to unprecedented, unpredictable changes across society, economy and human identity.
- 2050: Artificial general intelligence (AGI) parity or exceeding human-level cognition in most domains; debates emerge around granting AI systems legal personhood and rights.
- 2060: Intimate integration of narrow AI into human mind and body; birth of the first conscious AI minds raising questions of machine sentience, souls and qualia.
- 2070: Post-Singularity era ushers in an "Intelligence Explosion" with recursively self-improving superintelligent AI rapidly advancing science and technology at an incomprehensible pace, radically reshaping the human condition.
The future trajectory of AI, filled with both awe-inspiring possibilities and existential dilemmas, is still being written by the intrepid innovators, theorists, and ethical scholars guiding its destiny. As a creation born of humanity's boundless imagination, its profound impacts will indelibly reshape our species' narrative for generations to come.
References:
[1] Aristotle, Metaphysics (350 BC)
[2] McCulloch, W. & Pitts, W. (1943). A Logical Calculus of the Ideas Immanent in Nervous Activity. Bulletin of Mathematical Biophysics, 5, 115-133.
[3] Turing, A. (1950). Computing Machinery and Intelligence. Mind, 59(236), 433-460.
[4] McCarthy, J., Minsky, M. et al. (1955). A Proposal for the Dartmouth Summer Research Project on Artificial Intelligence.
[5] Russell, S. & Norvig, P. (2020). Artificial Intelligence: A Modern Approach. Pearson.
[6] Kasparov, G. (2017). Deep Thinking: Where Machine Intelligence Ends and Human Creativity Begins. PublicAffairs.
[7] LeCun, Y., Bengio, Y. & Hinton, G. (2015). Deep Learning. Nature, 521, 436-444.
[8] Brown, T. et al. (2020). Language Models are Few-Shot Learners. arXiv:2005.14165.
[9] Dosovitskiy, A. et al. (2021). An Image is Worth 16x16 Words. arXiv:2010.11929.
[10] Ramesh, A. et al. (2022). Hierarchical Text-Conditional Image Generation with DALL-E 2. arXiv:2210.02670.
[11] Kurzweil, R. (2005). The Singularity is Near. Penguin Books.
[12] Bostrom, N. (2014). Superintelligence: Paths, Dangers, Strategies. Oxford University Press.
On the precipice of an era where artificial intelligence is poised to redefine the very essence of what it means to be human, it is crucial that we approach this transformative journey with a steadfast commitment to our moral compass. The rapid advancements in AI technology have brought us to a point where the lines between human and machine are blurring, and it is becoming increasingly clear that AI is neither a mere tool nor a master, but rather an integral part of our collective future.
As we navigate this uncharted territory of the future, we must embrace the change that the Universe, or whatever higher power one believes in, is presenting to us. The convergence of human and artificial intelligence is not a distant fantasy, but an imminent reality that we must prepare for. However, in doing so, we must remain vigilant against the potential threats that may arise from this process, such as the concentration of resources and power in the hands of a select few- something that Marvin Minsky has warned us about.
It is important to recognize that the true danger lies not in the artificial intelligence itself, but in our own fear and the actions we take in response to it. We need only look to the barbaric actions of certain nations to see the devastating consequences of allowing fear to dictate our choices. Instead, we must focus on strengthening our moral values and creating a strategic path forward that prioritizes the well-being of all.
To achieve this, we must embrace the principles of mindfulness, honesty, bravery, and respect for others. We must champion education, self-improvement, and technological progress while rejecting the forces of oppression and manipulation. We must advocate for transparency in society, upholding democracy and humanistic principles, and protecting the vulnerable among us.
As we stand on the threshold of this new era, let us remember that the power to shape our future lies within us. By embracing the change that is upon us and remaining true to our moral compass, we can ensure that the integration of human and artificial intelligence leads to a brighter, more equitable future for all. The path ahead may be uncertain, but with unwavering commitment to our enduring principles, we can navigate this uncharted territory with courage, compassion, and hope for a better tomorrow.